CORE Recommender is a plugin for repositories, journals and web interfaces that provides suggestions on relevant articles to the article a user is looking for. The source of recommended data is the base of CORE, which consists of over 25 million full texts from CORE. Today we have interviewed George Macgregor, Scholarly Publications & Research Data Manager at the University of Strathclyde, responsible for the Strathprints institutional repository. Read about his experience of using CORE Recommender on the Jisc Research blog.
Tag: recommender
CORE update for January to March 2020
The first quarter of 2020 was a highly productive period for CORE in terms of growing and developing our products. Details about these and more news can be found below.
CORE is ready to release a premium version of the Repository Dashboard
The CORE team has developed a premium edition of the CORE Repository Dashboard, with a particular focus being on the development of features that support compliance assessment with the REF 2021 Open Access Audit.
The new CORE Repository Dashboard contains a brand new REF compliance and DOI enrichment tabs. This service has been developed to support Higher Educational Institutions (HEIs) with repositories. More specifically, repository managers, research administrators, etc. The interface offers valuable technical information and statistics. Users can benefit from the tool by accessing information that will help them to improve the harvesting of their repository outputs and increase the visibility of their content. The premium edition is currently available to a limited number of users and we plan to expand to all interested institutions in the future. The release of the new version will be announced through a CORE blog-post.
CORE Recommender installation for DSpace
In this blog post, Alex Efimov, Staff Engineer at the Research Office of the Ural Federal University, gives a step by step guide on how to install the CORE Recommender on DSpace. The post is also available in Russian.
The CORE Recommender is a large and complex service, while its main purpose is to advance a repository by recommending similar articles. This blog post reviews only the plugin for a dspace/jspui based repository. The source of recommended data is the base of CORE, which consists of metadata descriptions and full texts. In addition, this plugin can recommend articles from the same repository as well.
Are you an iOS user? Access scientific articles in your device without hitting a paywall
Another year has passed and left a lot of good news, investigations and developments for CORE. Today we would like to tell you about one of them – Open Access (OA) Helper, an application developed for iOS mobile devices by Claus Wolf. We asked Claus to tell us how he came up with the OA Helper and here is what he answered.
When, where and why did you decide to develop OA Helper app?
In October 2018, I learned about how open access discovery services connect users to legal Open Access copies of otherwise paywalled articles. The available plugins weren’t available for Safari, my preferred browser, so I decided to give creating one a try.
CORE users can now read articles directly on our site
We are happy to announce the release of CORE Reader, which provides a seamless experience for users wishing to read papers hosted by CORE. In this post, we provide an overview of what is new and we encourage you to follow this development as new functionalities in the reader are on our roadmap.
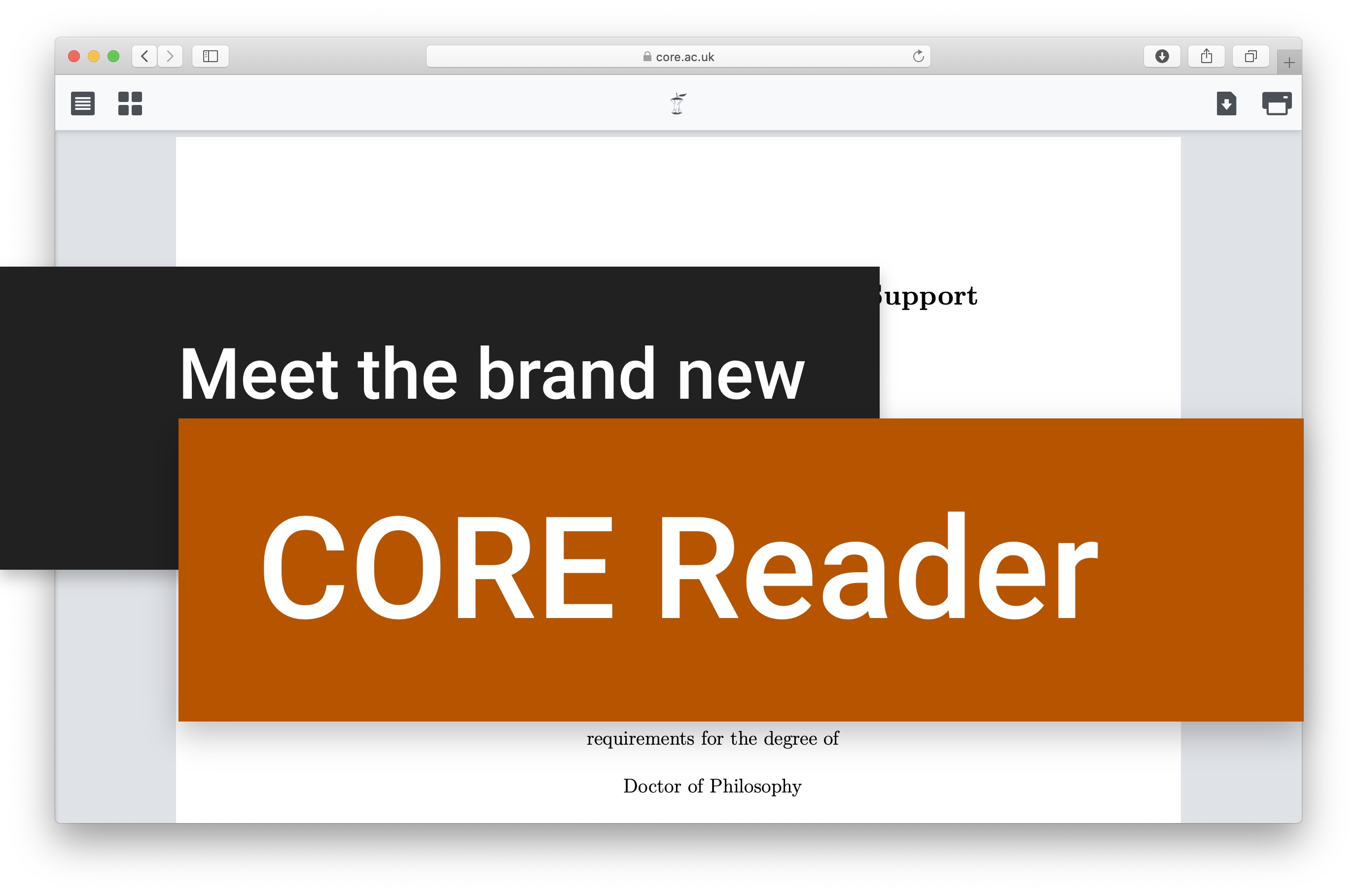
At the beginning of this project, there was a reflection that most open access services do not yet provide a rich user experience for reading research papers. Determined to change this, we originally started looking at whether CORE could render research papers as HTML, as has recently become trendy across publisher platforms. While such rendering remains to be one of the ultimate goals, we realised that this could only be achieved for a small fraction of documents in CORE. More specifically, those that the data provider offers in machine readable formats, such as LaTeX or JATS XML. While we want to encourage more repositories to support such formats (and this remains to be a Plan S recommendation), we wanted to improve the reading experience for all of our users across all of our content.
Releasing a new CORE Discovery browser extension
CORE Discovery helps users find freely accessible copies of research papers that might be behind a paywall on the publisher’s website. It is backed by our huge dataset of millions of full text open access papers as well as content from widely used external services beyond CORE. The tool not only provides state-of-the-art coverage of freely available content, it is the only discovery service which:
- delivers state-of-the-art performance compared to other discovery tools in terms of both content coverage (finding a freely available copy when it is available) and precision (reliably delivering a free copy of the paper on success);
- is run by researchers for researchers (as opposed to companies);
- has the best grip on content from the global network of open repositories;
- can deliver to readers other relevant freely available research papers even in situations where a freely available version is not available from anywhere on the web.
To satisfy the needs of CORE users, the world’s largest global aggregator of open access research papers now helps users access articles of their interest. Generally, discovery tools can find typically free copies of papers for about 15%-30% of published documents (slide 11). This means that in more than 70% of cases, they don’t bring to the user anything useful. CORE Discovery can offer the user relevant documents even in situations where other discovery tools are not successful. What distinguishes CORE Discovery from other discovery services on the market is that it does not stop when an open access version is not available, but always aims to offer related open access articles to the end user.
LA Referencia integrates CORE Recommender in its services
LA Referencia – an aggregator of research papers from Latin America collaborates with CORE – a scholarly communications infrastructure that provides access to the world’s largest collection of open access research publications, acquired from a global network of repositories and journals.
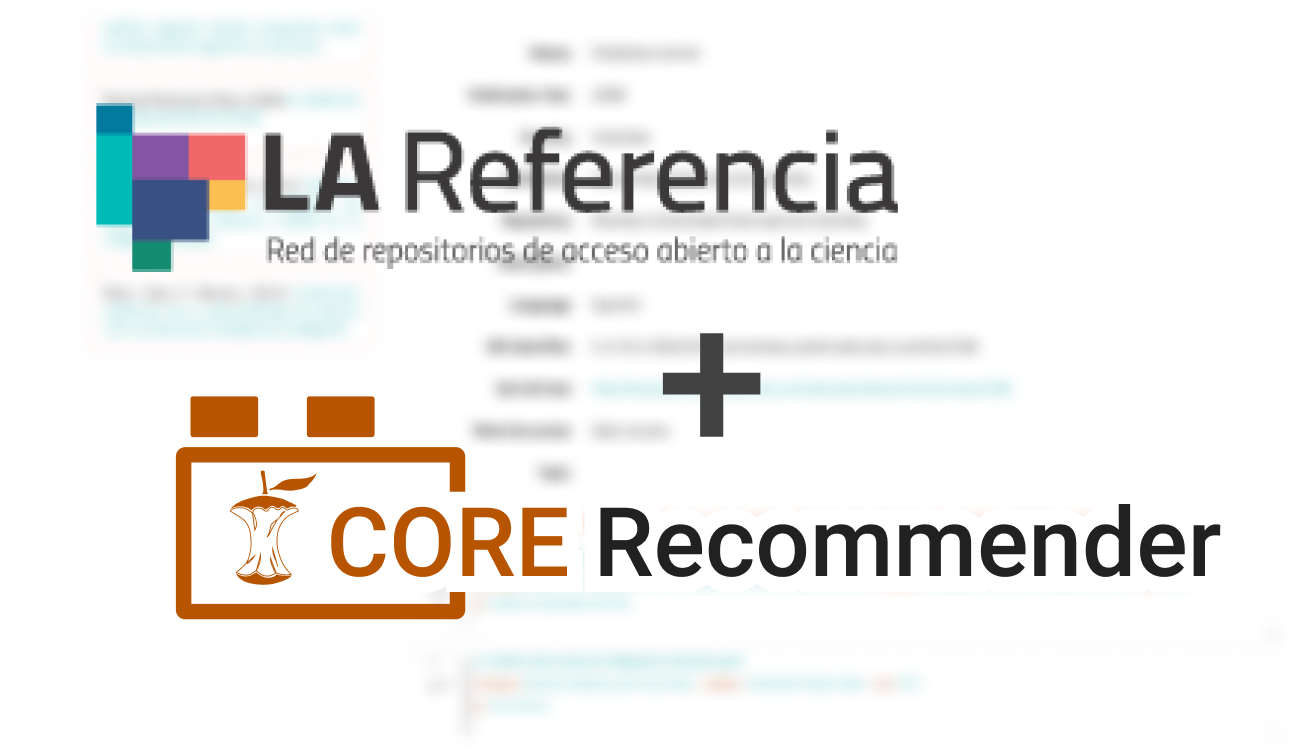
CORE Recommender is now integrated within LA Referencia, allowing users to discover similar articles from across a network of thousand open access data providers. CORE Recommender acts as a gate to millions of open access research papers, suggesting relevant articles where the full text is guaranteed to be openly available. Moreover, the recommender delivers to users only free to read materials, i.e. materials that can be accessed without hitting a paywall.
Implementing the CORE Recommender in Strathprints: a “whitehat” improvement to promote user interaction
This guest blog post briefly reviews why the CORE Recommender was quickly adopted on Strathprints and how it has become a central part of our quest to improve the interactive qualities of repositories.
Back in October 2016 my colleagues at the CORE Team released their Recommender plugin. The CORE Recommender plugin can be installed on repositories and journal systems to recommend similar scholarly content. On this very blog, Nancy Pontika, Lucas Anastasiou and Petr Knoth, announced the release of the Recommender as a:
…great opportunity to improve the functionality of repositories by unleashing the power of recommendation over a huge collection of open-access documents, currently 37 million metadata records and more than 4 million full-text, available in CORE*.
(* Note from CORE Team: the up-to-date numbers are 80,097,014 metadata and 8,586,179 full-text records.).
When the CORE Recommender is deployed a repository user will find that as they are viewing an article or abstract page within the repository, they will be presented with recommendations for other related research outputs, all mined from CORE. The Recommender sends data about the item the user is visiting to CORE. Such data include any identifiers and, where possible, accompanying metadata. The CORE response to the repository then delivers CORE’s content recommendations and a list of suggested related outputs are presented to the user in the repository user interface. The algorithm used to compute these recommendations is described in the original CORE Recommender blog post but is ultimately based on content-based filtering, citation graph analysis and analysis of the semantic relatedness between the articles in the CORE aggregation. It is therefore unlike most standard recommender engines and is an innovative application of open science in repositories.
Needless to say, we were among the first institutions to proudly implement the CORE Recommender on our EPrints repository. The implementation was on Strathprints, the University of Strathclyde’s institutional repository, and was rolled out as part of some wider work to improve repository visibility and web impact. The detail of this other work can be found in a poster presented at the 2017 Repository Fringe Conference and
a recent blog post
Recommender sunset period
As a result, it means that we will stop supporting old versions of the recommender. If you installed the recommender on or before the 10th October 2016, you will need to upgrade.
Any old version of the recommender will cease to work on Monday 20th February 2016.
- How do I know if I need to upgrade?
In EPrints, visit ‘Admin’, ‘System Tools’, ‘Eprints Bazaar’. In the list of installed plugins, if you see ‘CORE Widget’ or version 0.x.x, then you will need to upgrade. For example, if you see the following information, you will need to upgrade:
Notice how the title is CORE Widget and the website link is core.kmi.open.ac.uk.
If you are on the latest version, you will see the name as ‘CORE Recommender’, the version will be 1.x.x. An example of this is:
Here, the title is CORE Recommender and has a link to our new website address https://core.ac.uk.
For non-EPrints installations:
We previously offered a Javascript installation process. The best way to know if you need to upgrade is if the API key you are using is longer than 20 characters. If you are still unsure, please get in touch.
- Can I use my old API key using the upgraded recommender?
No, you will need to register for a recommender ID. Your existing API key will continue to be valid for our API v2 requests.
If you need any help with upgrading or have any further questions, please contact us or write a comment below. We’ll be happy to help.
CORE Recommender
The CORE team is thrilled to announce the release of a new version of our recommender; a plugin that can be installed in repositories and journal systems to suggest similar articles. This is a great opportunity to improve the functionality of repositories by unleashing the power of recommendation over a huge collection of open-access documents, currently 37 million metadata records and more than 4 million full-text, available in CORE.
Recommender systems and the CORE Plug-In
Typically, a recommender tracks a user’s preferences when browsing a website and then filters the user’s choices suggesting similar or related items. For example, if I am looking for computer components at Amazon, then the service might send me emails suggesting various computer components. Amazon is one of the pioneers of recommenders in the industry being one of the first adopters of item-item collaborative filtering (a method firstly introduced in 2001 by Sarwar et al. in a highly influential scientific paper of modern computer science).
Over the years, many recommendation methods and their variations have been suggested, evaluated both by academia and industry. From a user’s perspective, recommenders are either personalised, recommendations targeted to a particular user, based on the knowledge of the user’s preferences or past activity, or non-personalised, recommending the same items to every user.
From a technological perspective, there are two important classes of recommender systems: collaborative filtering and content based filtering.
1. Collaborative filtering (CF):
Techniques in this category try to match a user’s expected behaviour over an item according to what other users have done in the past. It starts by analysing a large amount of user interactions, ratings, visits and other sources of behaviour and then builds a model according to these. It then predicts a user’s behaviour according to what other similar users – neighbour users – have done in the past – user-based collaborative filtering.
The basic assumption of CF is that a user might like an unseen item, if it is liked by other users similar to him/her. In a production system, the recommender output can then be described as, for example, ‘people similar to you also liked these items.’
These techniques are now widely used and have proven extremely effective exploratory browsing and hence boost sales. However, in order to work effectively, they need to build a sufficiently fine-grained model providing specific recommendations and, thus, they require a large amount of user-generated data. One of the consequences of insufficient amount of data is that CF cannot recommend items that no user has acted upon yet, the so called cold-items. Therefore, the strategy of many recommender systems is to expose these items to users in some way, for example either by blending them discretely to a home page, or by applying content-based filtering on them decreasing in such way the number of cold-items in the database.
While CF can achieve state-of-the-art quality recommendations, it requires some sort of a user profile to produce recommendations. It is therefore more challenging to apply it on websites that do not require a user sign-on, such as CORE.
2. Content-based filtering (CBF)
CBF attempts to find related items based on attributes (features) of each item. These attributes could be, for example the item’s name, description, dimensions, price, location, and so on.
For example, if you are looking in an online store for a TV, the store can recommend other TVs that are close to the price, screen size, and could also be similar – or the same – brand, that you are looking for, be high-definition, etc. The advantage of content-based recommendations is that they do not suffer from the cold-start problem described above. The advantage of content-based filtering is that it can be easily used for both personalised and non-personalised recommendations.
The CORE recommendation system
There is a plethora of recommenders out there serving a broad range of purposes. At CORE, a service that provides access to millions of research articles, we need to support users in finding articles relevant to what they read. As a result, we have developed the CORE Recommender. This recommender is deployed within the CORE system to suggest relevant documents to the ones currently visited.
In addition, we also have a recommender plugin that can be installed and integrated into a repository system, for example, EPrints. When a repository user views an article page within the repository, the plugin sends to CORE information about the visited item. This can include the item’s identifier and, when possible, its metadata. CORE then replies back to the repository system and embeds a list of suggested articles for reading. These actions are generated by the CORE recommendation algorithm.
How does the CORE recommender algorithm work?
Based on the fact that the CORE corpus is a large database of documents that mainly have text, we apply content-based filtering to produce the list of suggested items. In order to discover semantic relatedness between the articles in our collection, we represent this content in a vector space representation, i.e. we transform the content to a set of term vectors and we find similar documents by finding similar vectors.
The CORE Recommender is deployed in various locations, such as on the CORE Portal and in various institutional repositories and journals. From these places, the recommender algorithm receives information as input, such as the identifier, title, authors, abstract, year, source url, etc. In addition, we try to enrich these attributes with additional available data, such as citation counts, number of downloads, whether the full-text available is available in CORE, and more related information. All these form the set of features that are used to find the closest document in the CORE corpus.
Of course not every attribute has the same importance as others. In our internal ranking algorithm we boost positively or negatively some attributes, which means that we weigh more or less some fields to achieve better recommendations. In the case of the year attribute, we go even further, and apply a decay function over it, i.e. recent articles or articles published a couple of years ago get the same boosting (offset), while we reduce the importance of older articles by 50% every N years (half-life). In this way recent articles retain their importance, while older articles contribute less to the recommendation results.
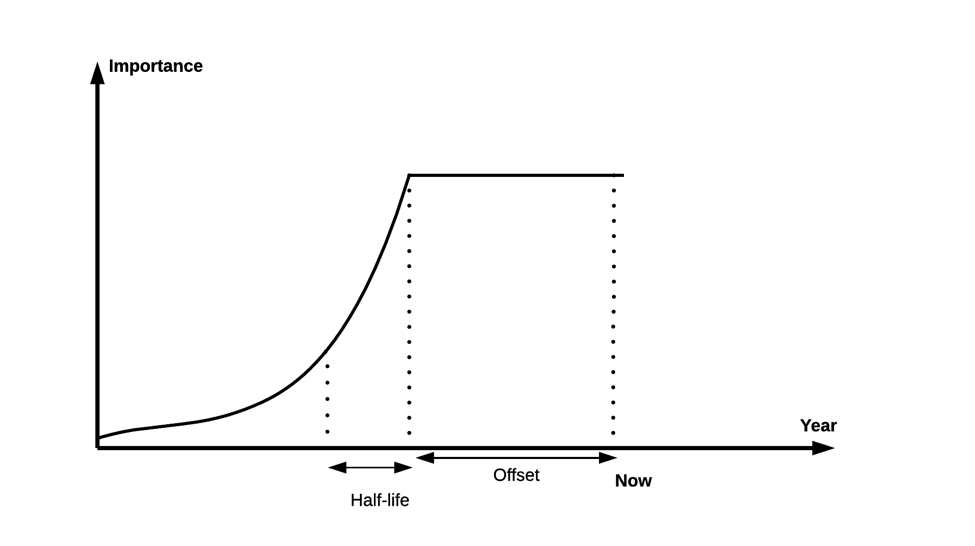
Someone may ask:
how do you know which weight to put in each field you are using? How did you come up with the parameters used in the decay function?